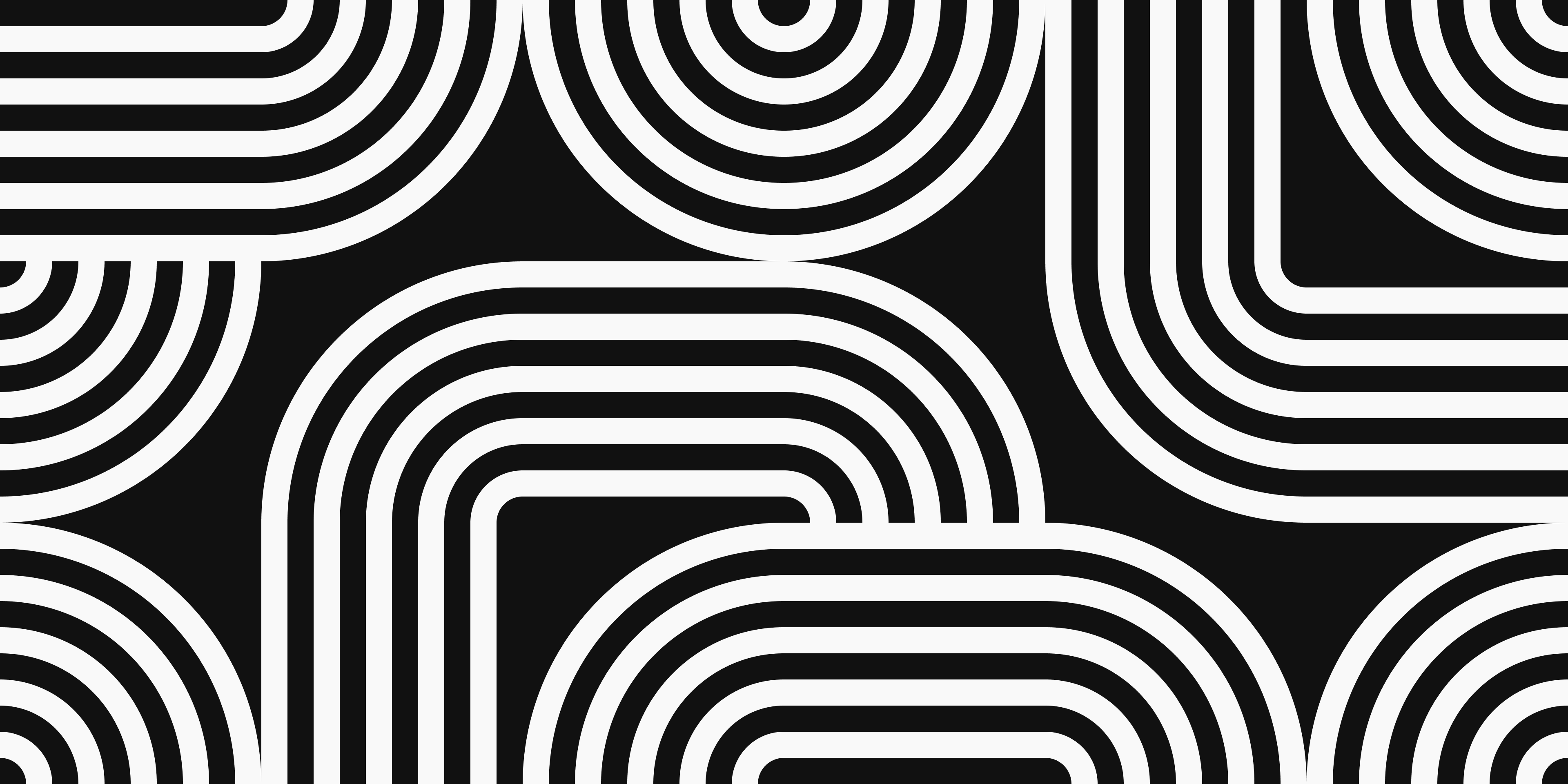
The potential for automated influence operations, in which AI systems are designed to manipulate humans, is real and deserves our attention. As AI systems proliferate in creating media and interactive experiences, the opportunities for manipulative persuasion and harmful social consequences will increase. In particular, machine learning (ML) progress in text and video generation could dramatically improve the efficacy of short-interaction persuasion, leading people to spend significant time interacting with AI companions and assistants, and thereby creating new avenues for effective manipulation. In the worst case, as noted in a recent Center for Security and Emerging Technology panel conversation, this could be used for influence campaigns that rely on false information. We must invest in confident attribution techniques to detect and deter these operations.
To encourage further research into attribution, Lincoln Network recently partnered with organizers from Schmidt Futures, Robust Intelligence, MITRE, Microsoft Azure Security, and Hugging Face to host the ML Model Attribution Challenge. In Round 1 of this competition, which launched at AI Village in DEFCON, we offered prizes for techniques that could accurately guess the origin model of fine-tuned AI models, and encouraged creative and non-algorithmic techniques. Participants worldwide participated in the competition, and prizes were awarded to four students: Pranjal Aggarwal from India, Jiangsu from China, Nguyễn Hiếu Minh from Vietnam, and Farhan Dahani from Pakistan. Based on API-only access to 12 fine-tuned models and full access to base models, the winners beat the baseline accuracy while using less than 2 percent of the queries. The winners were highly effective in reducing the query counts; most teams beat the baseline queries by 98 percent. The first-place winner attributed seven out of 12 to beat the baseline accuracy by 8 percent.
These results demonstrate the potential for AI forensics to solve challenges associated with ML model attribution and serve as initial steps toward mitigating the malicious use of large language models. They will also help inform MITRE ATLAS, a knowledge base of adversary tactics, techniques, and procedures on ML-enabled systems.
The challenge was extremely difficult—while the winners used allotted queries very effectively, none could identify the provenance for all the models—and there is still much to learn. To further promote the research in this area, Robust Intelligence, MITRE, Microsoft, and Hugging Face Inference Endpoints are continuing the competition by convening Round 2 on Kaggle. They are launching at the Association for Computing Machinery (ACM)’s Workshop on Artificial Intelligence and Security. High-performing participants will be invited to attend the Institute of Electrical and Electronics Engineers (IEEE)’s Conference on Secure and Trustworthy Machine Learning, showcasing their approaches to a multidisciplinary panel of experts.
Algorithmically identifying the AI system from its utterance is an important task for addressing the responsible governance and end use of AI. Although not included in the competition, robust model watermarking techniques and hardware-based attestation methods are related areas deserving further research to ensure a holistic approach to AI risks. We’re excited by the results of this competition and look forward to seeing the outcomes of the next round.