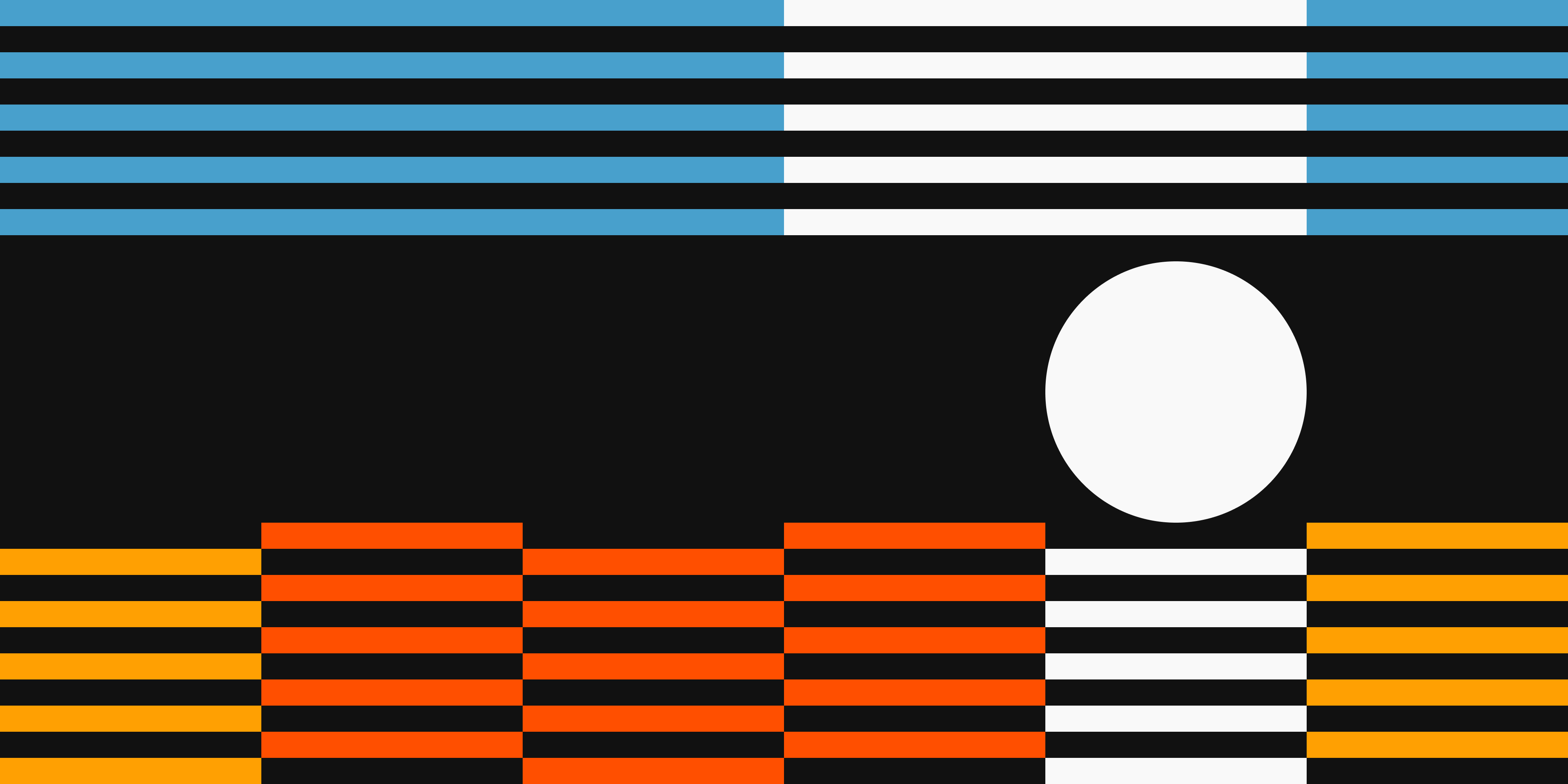
Today, I submitted a comment to the Office of Management and Budget regarding the draft memorandum titled Advancing Governance, Innovation, and Risk Management for Agency Use of Artificial Intelligence. Click here to download a pdf of the comment.
Thank you for the opportunity to respond to the Office of Management and Budget’s request for comment on the draft memorandum titled Advancing Governance, Innovation, and Risk Management for Agency Use of Artificial Intelligence (AI). My name is Samuel Hammond, senior economist for the Foundation for American Innovation. FAI is a group of technologists and policy experts focused on developing technology, talent and ideas to support a freer and more abundant future.
My research at FAI focuses on the second order effects of technologies like Artificial Intelligence on our institutions. By second order, I mean not only what an AI system can do on its own, but what is likely to result as AI capabilities diffuse throughout the economy. These second order effects are all important, as the history of transformative technologies – from the printing press to the industrial revolution to the internet – is also a history of equally transformative changes to government.
I call the tendency to neglect the second order effects from technology the Horseless Carriage Fallacy, as if the advent of the automobile merely replaced horse drawn carriages while holding everything else constant. In reality, the automobile changed virtually everything, radically reshaping American institutions and economic geography.
Artificial Intelligence will do the same. The question is whether governments will keep up and adapt, or be stuck riding horses while society whizzes by in the digital equivalent of a race car. The risks from adopting AI in government must therefore be balanced against the greater risks associated with not adopting AI proactively enough – a theme I explore in my essay series, AI and Leviathan.
Avoiding duplication, fragmentation, and anachronism
It’s within this context that I applaud the White House’s steps to promote the use of AI in government through the Executive Order on “Safe, Secure, and Trustworthy Development and Use of Artificial Intelligence.” It does this in a variety of ways, including through the formation of an interagency council at OMB to coordinate the use and development of AI in concert with new Chief AI Officers at each agency.
It is difficult in the abstract to know whether this framework will accelerate AI adoption in government or whether new layers of oversight will simply slow things down. As OMB begins implementing the Executive Order through this draft memorandum, it is thus imperative to retain the EO’s spirit of advancing AI in government by periodically evaluating whether the framework is proving effective in practice, and to create a process to adjust, revisit or amend the framework overtime.
Consider the establishment of Artificial Intelligence Governance Boards with oversight over AI issues at each agency. On the one hand, the Boards could expedite the adoption of AI by enabling a degree of central coordination. On the other hand, insofar as AI is increasingly embedded in every form of software, it is often hard to distinguish an AI system from ordinary information technology (IT). For example, techniques rooted in generative AI are increasingly used as an alternative method of data compression, enabling ultra low-bandwidth video conferencing. In the limit, some AI researchers are even exploring the use of generative AI as a general-purpose computing paradigm to replace conventional operating systems. Thus, as the line between AI systems and traditional IT systems blurs, the official definitions used to delineate “AI” from “non-AI” circa 2023 could become anachronistic in short order.
Whether the framework is successful will depend on whether the Boards and AI Officers are primarily incentivized to accelerate the adoption of advanced AI systems or to police phantom risks, like bias or discrimination, that aren’t unique to AI. As it stands, OMB was already two years late and well past its statutory deadline to issue AI guidance to agencies as required by the AI in Government Act of 2020. Before entrenching an additional layer of process, OMB should assess the factors that inhibited this timely issuance of guidance in its recent past, and use the enactment of this draft memorandum as an opportunity to correct or otherwise streamline those factors going forward.
The dangers from entrenching slow or duplicative approval processes is hard to overstate. FedRAMP, for instance, was created to overcome this problem in the procurement of cloud services. As a government-wide compliance program, agencies can transact with FedRAMP-approved providers knowing that they meet a standardized level of security. The most common AI services should follow the same model and be evaluated and authorized for use government-wide, letting Chief AI Officers and Governance Boards focus on overseeing bespoke or ad hoc AI systems for specific agency needs. This could be achieved by integrating NIST’s AI RMF into the FedRAMP approval process, as is already the case with NIST’s standards on security and privacy (SP 800-53). Insofar as many AI systems are and will continue to be accessed via the cloud, piggybacking on FedRAMP is only logical.
Winning the institutional arms race
The case for aggressive adoption of AI in government comes down to the arms race between AI and our institutions. This is most obvious in the arena of cybersecurity. As AI lets hackers and other bad actors level-up their capabilities, our cyber defenses will need to level-up at least as fast. And yet these dynamics extend far beyond cases of explicit AI misuse. Democratized access to AI lawyers could quickly overwhelm the court system, for instance, just as expert AI tax accountants could soon democratize the ability for individuals and businesses to minimize or complexify their tax liability.
These vectors of attack don’t constitute misuses of AI at all, but rather appropriate use at an unprecedented scale. In the near future, for instance, AI agents will likely fully automate the process of filing and appealing a FOIA request. Any information that can be requested will be, necessitating the adoption of e-discovery systems to allow AI agents to automatically review and fulfill the request on the government’s end. The final equilibrium could make for a far more transparent and efficient government, but in the interim, having many more requests for information than an agency has capacity to fulfill could cause a de facto Denial of Service attack. Similar such “attacks” are likely possible across any number of public venues or services, from AI generated regulatory comments, to the potential illegibility of the sheer volume of economic activity unlocked by AGI.
Even the most productive uses of AI will increase the throughput demand on our administrative agencies by orders of magnitude. Just last week, Google DeepMind published an AI model that discovered 2.2 million new crystals and 380,000 new stable materials that could power future technologies. This represents nearly 800 years’ worth of new material science knowledge, achieved virtually overnight. Imagine what will happen when this same pace of discovery comes to medicine, as it almost surely will. In a typical year, the FDA approves around 50 new molecular entities for novel drugs. Could the FDA handle increasing this approval rate to 500, 5,000 or even 50,000 new molecules per year, unlocking centuries of progress in personalized medicine? The answer is clearly no, at least without adopting AI symmetrically.
The need for (and risks of) scalable governance
In every case, managing these growing throughput demands will require the federal government to not only adopt AI aggressively, but should force a rethink of the configuration of our administrative and regulatory agencies from the ground up. From broken procurement policies to the bureaucratic sclerosis engendered by slow and outdated administrative procedures, incremental reform is unlikely to suffice. We must modernize government at the firmware-level, or risk ubiquitous system failure and government becoming the primary bottleneck to AI’s enormous potential upside.
In short, as throughput demands on government increase, we will need to adopt AI-native governance models that scale. One highly scalable approach is the idea of Government as a Platform, drawing an analogy to multi-sided platform companies like Uber or AirBnB. Such platforms use reputation mechanisms, search and matching algorithms, and automatic dispute resolution systems to provide quasi-regulatory forms of market governance with unprecedented scale. Given progress in AI, similar platform-based approaches could be extended to other areas of regulatory oversight.
Consider the recent advances in the visual understanding exhibited by Large Multi-modal Models (LMMs) such as GPT4-Vision. Such models can be prompted to describe and interpret what they “see” in any given image or video scene. As these models continue to improve and overcome their current limitations (such as vulnerability to prompt injection), LMMs could thus unlock new, highly scalable forms of regulatory oversight. For example, imagine a near future where, instead of the USDA or OSHA sending agents to inspect commercial farms or work sites in-person, cameras were required to be installed on-site with LMMs fine-tuned to continuously monitor for food or workplace safety violations.
As the above hypothetical makes clear, the power of AI to scale regulatory compliance also scales potential forms of government surveillance. To preserve Americans’ right to privacy and strengthen public trust, any AI system that has surveillance as a dual-use must have privacy and civil liberty protection engineered into the technology itself.
A lesser appreciated risk with AI-enabled regulatory oversight is simply the extent to which all laws and regulations are implicitly calibrated to the expectation of imperfect information and an “acceptable” degree of non-enforcement. Consider the data showing that most drivers make “rolling stops” at stop signs the majority of the time – a practice that is technically illegal but which goes largely unenforced. Indeed, such laws were written with the expectation of deeply imperfect enforcement. If a car’s on-board computer used AI to automatically detect and report a driver every time they made a rolling stop, went 10 MPH over the speed limit, and so on, the de facto stringency of basic traffic laws would suddenly become oppressive indeed. Thus, to the extent AI dramatically reduces asymmetric information within a particular agency’s regulatory domain, regulators must be on guard that they are not strengthening the de facto stringency of existing laws or regulations in an analogously dramatic fashion. Nor is this risk hypothetical, as shown by the federal decision to prevent Tesla’s self-driving system from making rolling stops.
Reducing waste, expanding capacity
Earlier this year, researchers at OpenAI published a paper assessing the likely labor market impact of Large Language Models. They found 80% of the U.S. workforce could have at least 10% of their work tasks affected by the introduction of LLMs, with jobs like Accountants, Auditors, and Legal Secretaries facing an exposure rate of 100%. Many large companies have already begun downsizing or have plans to downsize, in anticipation of the enormous efficiency gains unlocked by emerging AI tools and agents.
Much of the work performed in government bureaucracies is especially low-hanging fruit for AI. OMB should thus undertake an analogous survey to discover which federal jobs are most exposed to AI, and to what extent legislation is needed to expedite new, AI-enabled models of governance. The goal should not be to downsize the federal bureaucracy per se, but rather to augment employee productivity and free up human resources for higher value uses, reducing waste and enhancing capacity simultaneously.
Take the FTC’s health care division, which employs around 30 attorneys to police competition across the entire U.S. health care industry. A day in the life of these attorneys looks like manually reading through tens of thousands emails subpoenaed from a pharma CEO as part of discovery. Yet today, with the right prompt engineering, one could feed those emails into a Large Language Model and simply ask it to find the most egregious examples of misconduct. This wouldn’t replace the attorney’s role in verifying what the AI discovers, but even with the imperfections of current models, it would nonetheless drive massive productivity gains – gains that we can be sure are being exploited by the private law firms on the other side.
At the same time, the same tools that can be used to enhance federal capacity can be further used to strengthen Congressional oversight. Most of the work and communications performed in any given agency is now machine readable. As agencies embrace AI internally, managers will be able to easily track and query the performance of their staff, automatically generating reports and work summaries from common document repositories. These same techniques could be used to expedite reports to Congress, and even enable near real-time monitoring of an agency’s activities. Call it Inspector General-GPT.
Innovating within government should mean more than plugging AI into an existing, outdated process and calling it a day. It will take true inventiveness and ambition. With appropriate urgency and coordination between the White House, OMB and Congress, we can forestall system failure by co-evolving our institutions with AI, enhancing public trust and saving taxpayers’ dollars in the process.